Fri, Mar 29, 2024, 09:00 – 10:00AM (PDT), 12:00 – 1:00PM (EST)
Abstract:
Cell-cell communication (CCC) is essential to how life forms and functions. However, accurate, high-throughput mapping of how expression of all genes in one cell affects expression of all genes in another cell is made possible only recently, through the introduction of spatially resolved transcriptomics technologies (SRTs), especially those that achieve single cell resolution. However, significant challenges remain to analyze such highly complex data properly.
On Mar 29 2024, Dr. Tao Wang will introduce a Bayesian multi-instance learning framework, spacia, to detect CCCs from data generated by SRTs, by uniquely exploiting their spatial modality. He will highlight spacia’s power to overcome fundamental limitations of popular analytical tools for inference of CCCs, including losing single-cell resolution, limited to ligand-receptor relationships and prior interaction databases, high false positive rates, and most importantly the lack of consideration of the multiple-sender-to-one-receiver paradigm. In his preprint, the team evaluated the fitness of spacia for all three commercialized single cell resolution ST technologies: MERSCOPE/Vizgen, CosMx/Nanostring, and Xenium/10X. Spacia unveiled how endothelial cells, fibroblasts and B cells in the tumor microenvironment contribute to Epithelial-Mesenchymal Transition and lineage plasticity in prostate cancer cells. Spacia was deployed in a set of pan-cancer datasets and showed that B cells also participate in PDL1/PD1 signaling in tumors.
Dr Wang’s work demonstrated that a CD8+ T cell/PDL1 effectiveness signature derived from spacia analyses is associated with patient survival and response to immune checkpoint inhibitor treatments in 3,354 patients. The team also revealed differential spatial interaction patterns between γδ T cells and liver hepatocytes in healthy and cancerous contexts. Overall, spacia represents a notable step in advancing quantitative theories of cellular communications.
Speaker:

Dr. Tao Wang runs a top computational immunology lab in UT Southwestern Medical Center. Statistics, informatics, medicine, and biology are the four integral pillars of Dr. Wang’s interdisciplinary research program. He has been working on mining public and in-house high throughput data to achieve a deeper understanding of the mechanisms of various human diseases, with a heavy emphasis on immunological diseases, and their diagnosis, prognosis, and treatment. Examples of Dr. Wang’s achievements include: (1) he created the pMTnet model for predicting the pairing between TCRs and T cell epitopes (Nature Machine Intelligence, 2021). (2) He is the first investigator to develop methodologies to integrate single T/B cell gene expression with TCR/BCR-sequencing data (publications in Nature Methods and Nature Machine Intelligence). (3) Dr. Wang developed advanced methodologies to study immune epitopes. He developed BepiTBR for accurate prediction of B cell epitopes (iScience, 2022). He developed a statistical methodology to consider the clonality structures of tumor neoantigens, which led to improved prognostic and predictive powers for cancer patients (Science Immunology, 2020). (4) He developed novel bioinformatics tools for analyses of spatial transcriptomics data (Nature Methods, 2022)
Strategic Alliance:
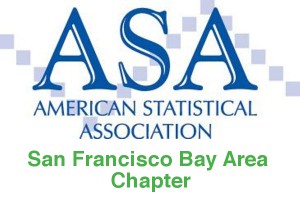
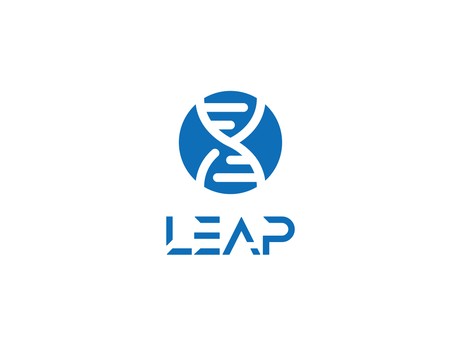
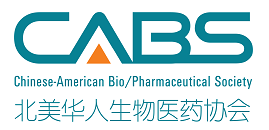
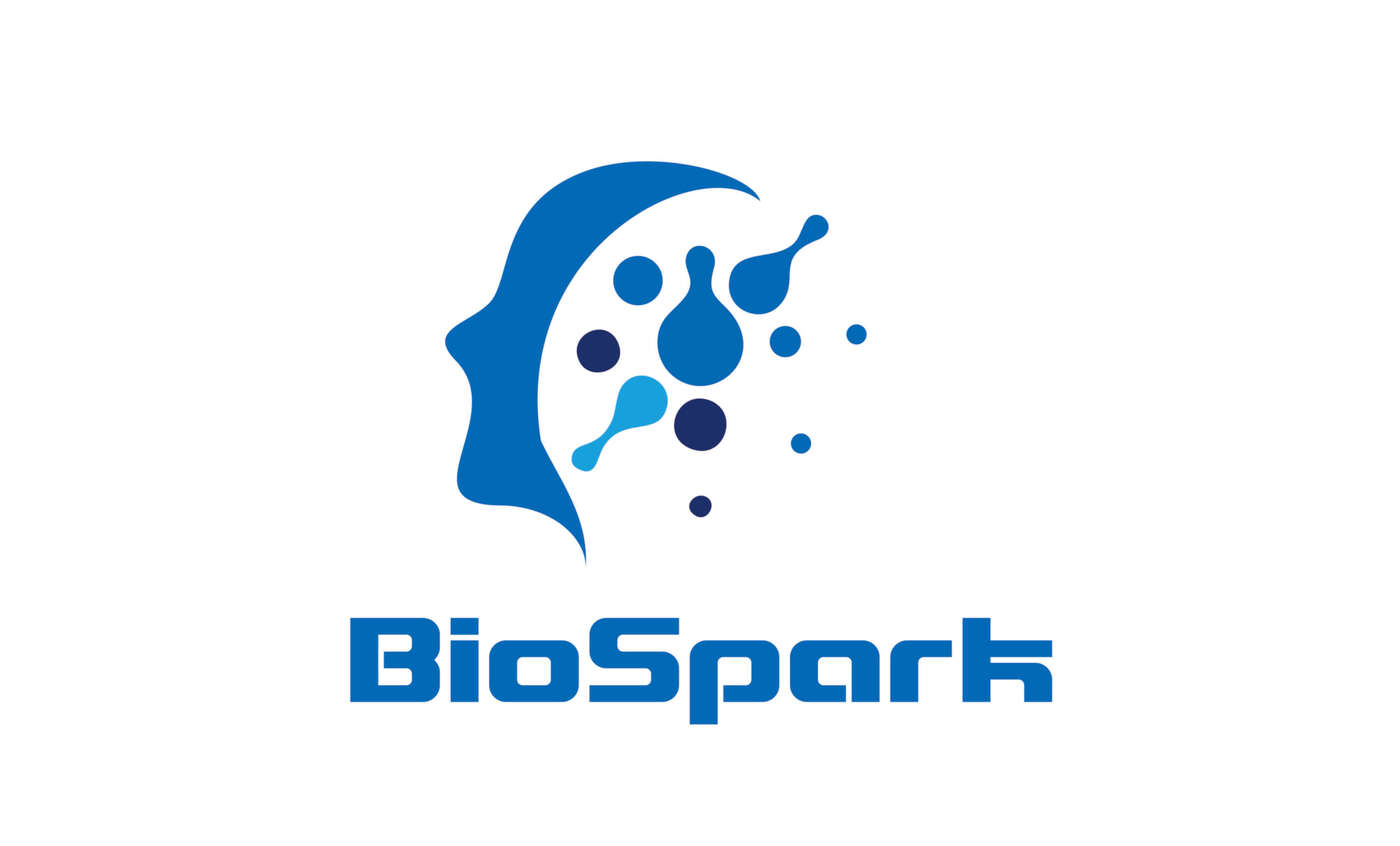