Fri, Jan 26, 2024, 09:00 – 10:00AM (PDT), 12:00 – 1:00PM (EST)
Speaker:

Dr. Le Lu leads the global Medical AI R&D efforts for Alibaba DAMO Academy since August 2021. Previously He had worked at PAII Inc., leading the division of Bethesda Research Lab, after more than five productive years at National Institutes of Health, Clinical Center, Radiology and Imaging Science Department, and from NVIDIA AI-Infra division and six years with Siemens Corporate Research and Siemens Medical Solutions USA. He is an IEEE Fellow (Computer Society) on medical imaging, AI and oncology imaging since 2021; also serves as an Associate Editor for IEEE Trans. Pattern Analysis and Machine Intelligence (IF: 24.3) since 2019, and IEEE Signal Processing Letters and Frontiers in Oncology. In 2007, he received his Ph.D. degree of Computer Science from Johns Hopkins University in the U.S.
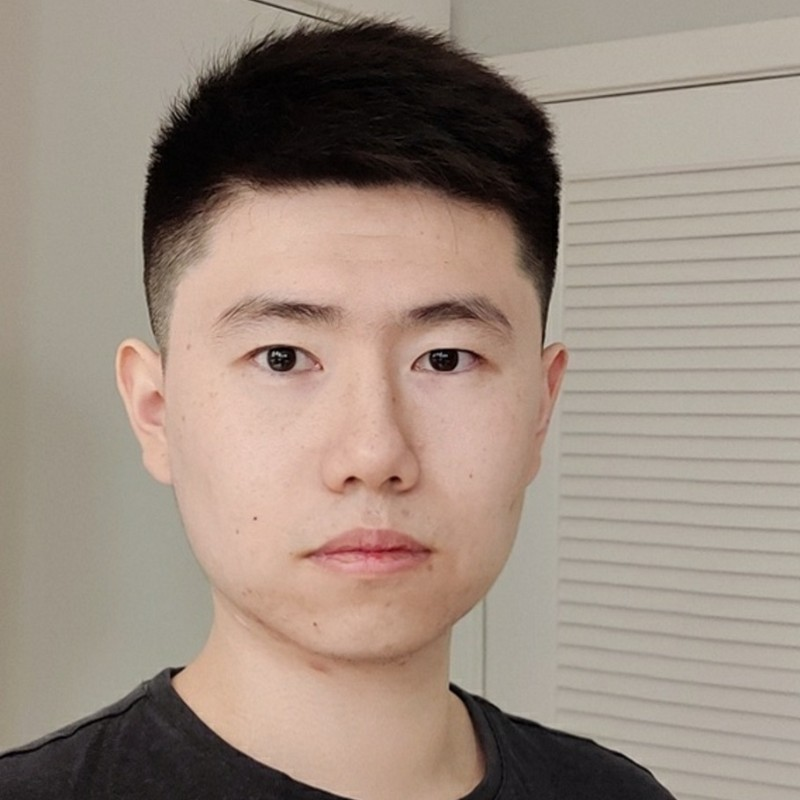
Dr. Yingda Xia is a research scientist at Alibaba DAMO Academy USA, working on real-world medical AI problems. He received his PhD from Johns Hopkins University, advised by Bloomberg Distinguished Professor Alan Yuille. His research interests are computer vision and medical image analysis. He has published 30+ peer-reviewed articles in top AI and medicine-related conferences and journals, such as Nature Medicine, Nature Communications, CVPR, ECCV, ICCV, NeurIPS, and MICCAI.
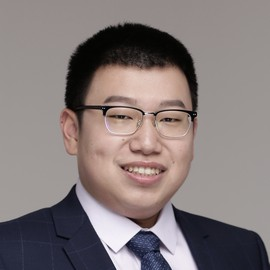
Dr. Jiawen Yao is a senior algorithm expert at Alibaba DAMO Academy. He obtained his PhD in Computer Science from University of Texas at Arlington under supervision from Dr. Junzhou Huang in 2019. His current research interests focus on developing computer vision and machine learning to improve the screening, diagnosis, and treatment recommendations in oncology for precision medicine. Those works are presented in top medical journals including Nature Medicine, Annals of Surgery, Radiology and Clinical Cancer Research (CCR).
Yingda's Talk
Title
Large-scale pancreatic cancer detection via non-contrast CT and deep learning
Abstract
Pancreatic ductal adenocarcinoma (PDAC), the most deadly solid malignancy, is typically detected late and at an inoperable stage. Early or incidental detection is associated with prolonged survival, but screening asymptomatic individuals for PDAC using a single test remains unfeasible due to the low prevalence and potential harms of false positives. Non-contrast computed tomography (CT), routinely performed for clinical indications, offers the potential for large-scale screening, however, identification of PDAC using non-contrast CT has long been considered impossible. Here, we develop a deep learning approach, pancreatic cancer detection with artificial intelligence (PANDA), that can detect and classify pancreatic lesions with high accuracy via non-contrast CT. PANDA is trained on a dataset of 3,208 patients from a single center. PANDA achieves an area under the receiver operating characteristic curve (AUC) of 0.986–0.996 for lesion detection in a multicenter validation involving 6,239 patients across 10 centers, outperforms the mean radiologist performance by 34.1% in sensitivity and 6.3% in specificity for PDAC identification, and achieves a sensitivity of 92.9% and specificity of 99.9% for lesion detection in a real-world multi-scenario validation consisting of 20,530 consecutive patients. Notably, PANDA utilized with non-contrast CT shows non-inferiority to radiology reports (using contrast-enhanced CT) in the differentiation of common pancreatic lesion subtypes. PANDA could potentially serve as a new tool for large-scale pancreatic cancer screening.
Jiawen's talk
Title
Effective Opportunistic Esophageal Cancer Screening Using Noncontrast CT Imaging
Abstract
Esophageal cancer is the second most deadly cancer. Early detection of resectable/curable esophageal cancers has a great potential to reduce mortality, but no guideline-recommended screening test is available. Although some screening methods have been developed, they are expensive, might be difficult to apply to the general population, and often fail to achieve satisfactory sensitivity for identifying early-stage cancers. In this work, we investigate the feasibility of esophageal tumor detection and classification (cancer or benign) on the noncontrast CT scan, which could potentially be used for opportunistic cancer screening. To capture the global context, a novel position-sensitive self-attention is proposed to augment nnUNet with non-local interactions. Our model achieves a sensitivity of 93.0% and specificity of 97.5% for the detection of esophageal tumors on a holdout testing set with 180 patients. In comparison, the mean sensitivity and specificity of four doctors are 75.0% and 83.8%, respectively. For the classification task, our model outperforms the mean doctors by absolute margins of 17%, 31%, and 14% for cancer, benign tumor, and normal, respectively. Compared with established state-of-the-art esophageal cancer screening methods, e.g., blood testing and endoscopy AI system, our method has comparable performance and is even more sensitive for early-stage cancer and benign tumor. Our proposed method is a novel, non-invasive, low-cost, and highly accurate tool for opportunistic screening of esophageal cancer.
Strategic Alliance:
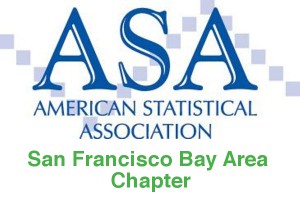
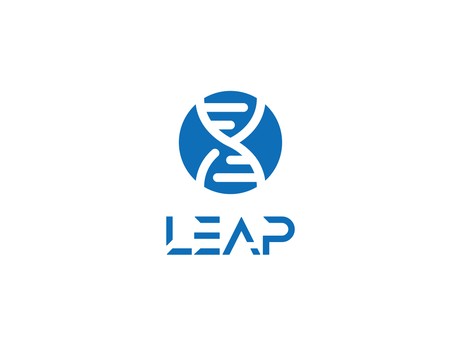
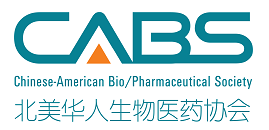
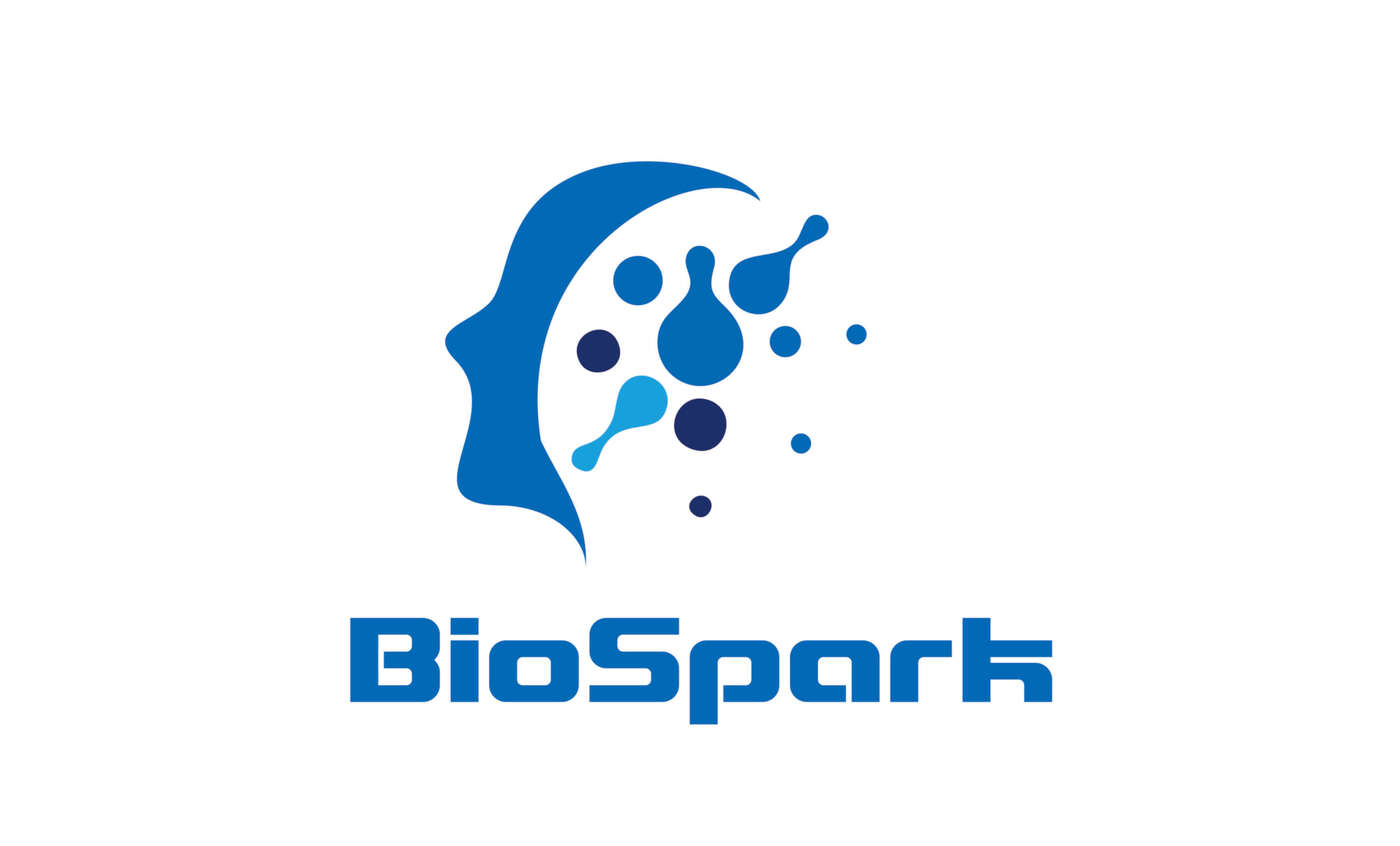